Unlock the power of automation
In this module, explore the benefits and challenges of robotic process automation (RBA) and intelligent automation (IA). Set realistic ROI and payback periods expectations in the application of automation.
Deciding to automate
Industry case studies show organisations typically pilot with an automation use-case that has high business value and low implementation complexity. They start with a basic RPA use case as a pilot and then progress to AI-powered use-cases. Intelligent automation use-cases demand a heightened capability to navigate increased complexity, acquire the necessary skills, and manage implementation costs effectively.
According to a Deloitte survey, 53% of organisations started their automation journey with RPA, most of them realising benefits within the first year.13 Gartner anticipates that operational expenses can be reduced by 30% using intelligent automation (IA) technologies.14 Despite the high return on investment (ROI), the implementation complexities of IA use-cases extend the organisation’s payback periods far beyond RPA use-cases.11,13
NSW Government business leaders and decision-makers should view ROI and payback as financial metrics and a strategic lever to drive efficiency and innovation. Conduct a detailed analysis to determine if automation aligns with your business strategies, considering the necessary investment in skills, scalability, and total cost of ownership (TCO). This approach will provide a clear understanding of expected ROI and payback time.
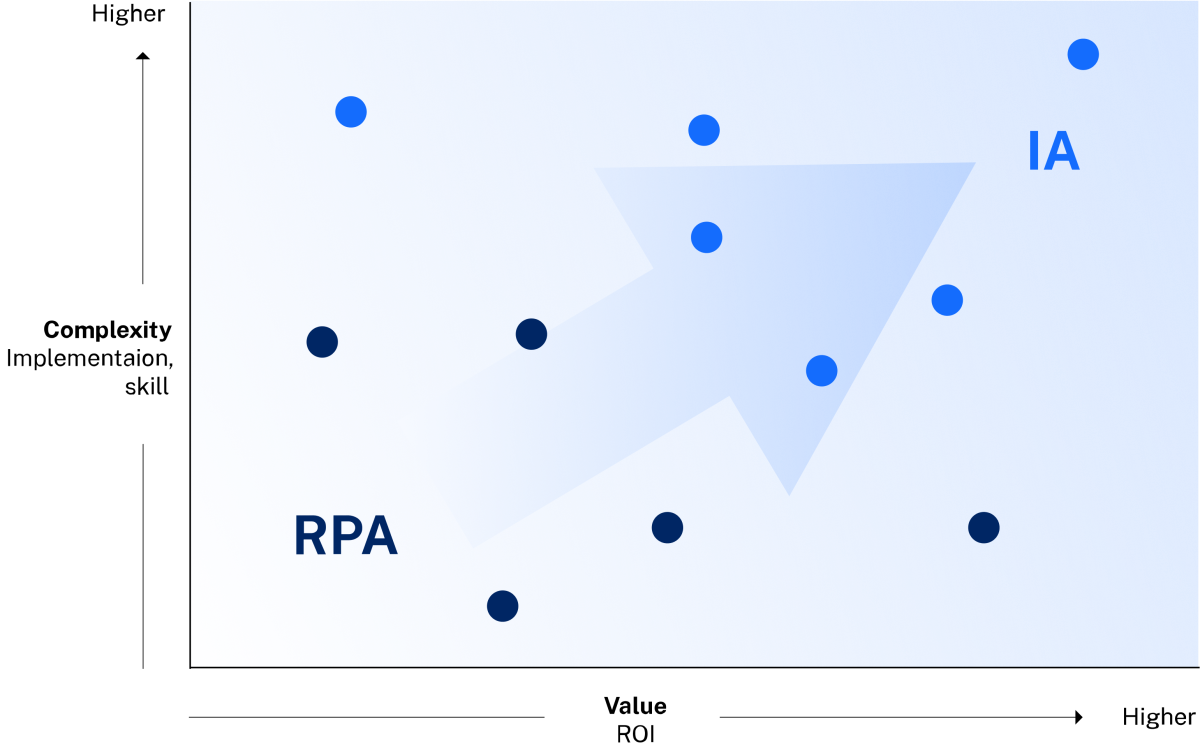
IA can offer higher cost reduction (+5%) and revenue increase (+9%) over RPA, but generally with longer payback periods (12-24 months instead of 6-12). RPA is often piloted first due to lower complexity, with 45% of organisations then scaling with IA.13
RPA: benefits and challenges
RPA streamlines rule-based manual tasks, improving efficiency, cutting costs, and boosting productivity. RPA solutions are scalable and can be quick to implement, resulting in rapid ROI. RPA is less effective with complex processes that involve unstructured data or require cognitive abilities. Challenges include upfront costs, ongoing maintenance, employee resistance, and security concerns. Expand below to unlock full list of RPA benefits and challenges.
Benefit | Description |
---|---|
Cost and time savings | Automating routine administrative tasks leads to reduced operational expenses. |
Operational efficiency | Streamlining workflows and processes, and optimising employees and resources utilisation improves overall efficiency. |
Accuracy and consistency | RPA ensures high accuracy in data entry and reduces errors associated with manual processes, ensuring consistency. |
Enhanced employee productivity | Frees up employees from repetitive tasks and reduce cycle times; leading to improved service delivery. |
Improved compliance | Enforces standardised processes, accurate records and reduces fraud probability, contributing to regulatory compliance. |
Scalability | RPA bots can be easily scaled up or down to accommodate changes in workloads. |
Fast implementation | In some use cases, RPA solutions can be implemented quickly, delivering immediate benefits. |
Quick ROI | RPA often yields quicker ROI due to lower complexity compared to Intelligent Automation. |
Legacy system integration | RPA can interact with legacy systems without the need for major system upgrades. |
Challenge | Description |
---|---|
Complex processes | RPA solutions struggle with complex processes that require human judgment. |
Limited cognitive abilities | RPA lacks the cognitive abilities of intelligent decision-making, such as understanding context, learning from experience, and making complex decisions. |
High upfront costs | Upfront implementation costs, including software, integration, and training. |
Dependency on structured data | RPA performs best with structured data; handling unstructured data can be challenging. |
Security concerns | Security risks can arise if proper controls are not in place. |
Change resistance | Employees may resist automation due to concerns about job security, changes in roles, or the need to learn new skills. |
Third-party liability | Misuse or improper licensing of third-party software or data may result in intellectual property infringement and legal action. |
IA: benefits and challenges
Intelligent Automation (IA) uses AI and machine learning for advanced decision-making and human-like interactions, empowering creative tasks and improving service delivery. IA requires expertise in AI and data, and faces challenges like biases, ethics, skilled workforce, regulatory compliance, and technical complexity. We recommend referring to the AI Assessment Framework (AIAF) to assess the AI benefits against the risks.3
Expand the table below to unlock a complete list of IA benefits and challenges.
IA Benefit | Benefit Description |
---|---|
Improved service delivery | Natural Language Processing (NLP) and chatbots enable human-like interaction while sentiment analysis provides customer behaviour and preferences insights, improving services delivery. |
Improved community satisfaction | Faster response times, personalised interactions, and reduced errors contribute to improved customer satisfaction. |
Informed decision-making | IA uses machine learning for advanced analytics and data-driven decision-making. |
End-to-end process optimisation | Tools such as process intelligence identifies bottlenecks and areas of improvement, contributing to end-to-end process optimisation. |
Adaptability and learning | IA systems adapt and improve processes over time through machine learning algorithms. |
Increased agility | IA allows organisations to respond quickly to changing market conditions. |
Innovation for improved services | Harnessing data-driving insights and advanced technologies enables innovative approaches to improving government services. |
Accessibility and inclusivity | Generative AI tools can improve the accessibility of an organisation’s services through language translation and summarising content. |
IA Challenge | Challenge Description |
---|---|
Data privacy | Handling sensitive data in IA applications may result in privacy issues. This requires careful planning, controls and ongoing monitoring. |
Data availability | Requires large volumes of high-quality data to train models effectively. |
High initial costs | Initial implementation costs for advanced technologies can be high. |
Innovation culture | A culture that supports multi-disciplinary teams and experimentation is required. |
Ready for use models | Some AI components may need extra development for safe use, including guardrails and consulting ethics, legal, and AI experts. |
Skilled professionals | Requires professionals with expertise in AI and data analytics. |
Integration complexities | Integrating IA with existing systems can be challenging due to its continuous learning and adaptation. |
Opportunity identification | Identifying and prioritising use-cases can be difficult when the technology is new. |
Vendor selection | Complexity of solutions, dynamic technology landscape, and the need for specialised expertise makes vendor selection challenging. |
Fair procedures | Ensuring outcomes are fair, evidence-based, legal, unbiased and ethical. The ability to justify decisions that affect rights. |
Auditability | More care is needed. Records and audit trails should be fully reviewed by oversight bodies. |
Transparency and accountability | Clarity in explaining AI decisions is required. As are ways to ensure accountability in AI operations. |
User trust and acceptance | Building trust and acceptance by ensuring AI systems are reliable, transparent, and aligned with human values. |
Elevated governance requirements | High standard of governance mandated to ensure the safe and responsible use of artificial intelligence. |